Common mistake series: probability sampling
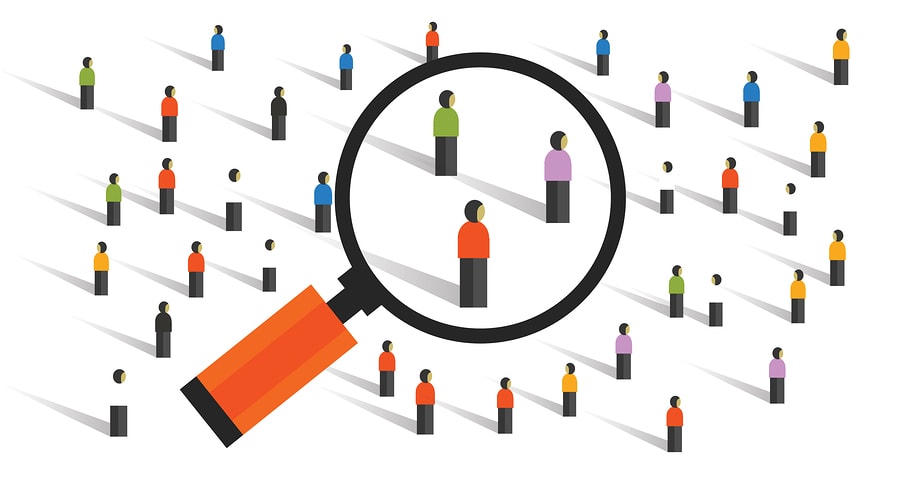
Written by: Dian Luthfiana Sufyan
image credit: masterofproject.com
“allow yourself to make mistakes, solely for the purpose of learning”
Common mistakes refer to the frequent inaccuracies made by researchers in selecting methodological aspects of submitted protocols. Most of the time, the common error emerges when dealing with two key areas (1) determining the appropriate sample size and (2) selecting subjects through sampling method.
This writing is the second and the last part of the common mistake series. The previous part of the series discussing sample size estimation can be retrieved here.
While the sample size contributes to the precision of research, the process of sample selection is crucial for establishing research validity. Both aspects are paramount to defining sample representativeness, as most novice researchers put more attention on sample size and overlook the sampling method.
In a nutshell, there are two main sampling methods, probability and non-probability sampling. Probability sampling incorporates randomization in the process that would generate an equal chance for the target population to be selected as samples, while non-probability involves a nonsystematic process. In this opportunity, the author will cover the probability sampling and use another chance to discuss the non-probability sampling.
As mentioned above, the involvement of randomization in probability sampling generates understanding that the population has the same chance to be recruited as sample. Thus, the use of probability sampling is expected to avoid bias. There are several types of probability sampling.
- Simple random sampling
Also called the golden standard of sampling techniques. This method involves direct randomization of target population. Thus, accessibility and availability of list of all subjects is a must. That is why, this method is only feasible when research involves a small-scope population, for instance, a study aims to investigate cognitive ability of English club members (N=100) in the writing band. Since the study will be conducted in an accessible scope and the list of members is available, then simple random sampling can be done.
- Stratified sampling
This method divides the target population into strata (stratification). Strata is a group with similar characteristics that are presumed to affect the estimated parameters of the study, such as age, gender, socioeconomic level, education, etc. After strata division, the researcher continues with simple random within strata. For example, a study for early detection of Type 2 Diabetes Mellitus will be carried out among students of one Senior High School. In this case, the strata can divide students based on their gender (male or female) or based on their age (16 or 17 or 18 years old).
- Cluster sampling
This method is considered when study settings involve a large size of population. Cluster sampling divides the target population based on geographical location into a list of clusters. A set of clusters is then further randomized to decide which location is involved in the study. Then, a list of individuals within clusters is randomly selected, just like simple random sampling. This method, also known as multistage sampling, involves the first step of cluster selection and the next step of subject recruitment. For instance, a study aims to determine the environmental factors of food choice among adult individuals living in Depok. It is going to be burdensome to have direct randomization from the list of all adults in Depok. In this case, a list of clusters can be made gradually by considering the population density across the Subdistrict (kecamatan), then making list of community units (RW) and researchers randomly pick up several RW, and recruits sample from eligible RW.
- Systematic sampling
As named, this method developed a systematic way to recruit samples, using a fixed interval. A certain formula of total population divided by sample size needed, is used to generate interval numbers. Hence, a list of target populations is necessary for reference. Further, to select the first sample, a random number generated by software should be multiplied by the interval. For example, a study aims to determine the prevalence of malnutrition and its associated factors among under-five (U5) children in Jakarta. The study has a list of eligible households which have under five children. For easy understanding, refer to the following formula.
Random number = 0.191 (generated by application with range of 0 to 1)
Interval = total population of U5 children/sample size needed
Sample 1 = 0.191 x interval
Sample 2 = Sample 1 + interval
Sample 3 = Sample 1 + (2 x interval)
Sample 4 = Sample 1 + (3 x interval)
etc
Mistakes often occur when researchers propose a quantitative study design but opt for non-probability sampling methods, typically purposive sampling, based solely on inclusion and exclusion criteria. While these criteria are essential in all studies, relying exclusively on purposive sampling can compromise sample representativeness and introduce bias, ultimately affecting the study's outcomes.
In summary, selecting the appropriate sampling method is crucial for safeguarding the validity of the study. Incorporating randomization is the preferred approach for quantitative research designs; however, researchers must also consider the feasibility of direct randomization. This requires a comprehensive understanding of the characteristics of the target population.
Dian Sufyan is a lecturer and researcher at Nutrition Department of UPNVJ, reviewer board member of Research Ethics Committee UPNVJ, 2020 SEAMEO RECFON affiliated researcher, DAAD alumnae and currently a Postgraduate Research Student at Human Nutrition Department, University of Glasgow
References
Alavi, M., Lohrasbi, F., Thapa, D. K., Biros, E., Lai, C., & Cleary, M. (2024). Achieving a representative sample in health research. Nurse Education in Practice, 78, 1–3. https://doi.org/10.1016/j.nepr.2024.103986
Ariawan, I. (2019). Sample size calculation and Sampling procedure. Presented on: Postgraduate Training on Survey Planning, 2 May 2019. SEAMEO RECFON, Faculty of Medicine, University of Indonesia: Jakarta.
Elfil, M., & Negida, A. (2019). Sampling methods in clinical research; an educational review. Archives of Academic Emergency Medicine, 7(1), 3–5.
Kementerian Kesehatan RI. (2021). Pemutakhiran Rumah Tangga (Updating RUTA) Studi Status Gizi Indonesia 2021. Presented on: Training for updating officials, 18 May 2021. Badan Penelitian dan Pengembangan Kesehatan, Kemenkes RI: Jakarta.
Lemeshow, S., Hosmer, D. W., Klar, J., & Lwanga, S. K. (1991). Adequacy of Sample Size in Health Studies. In World Health Organization (Vol. 47, Issue 1). John Wiley & Sons Ltd. https://doi.org/10.2307/2532527
Wretman, J. (2010). Reflections on probability vs nonprobability sampling. Official Stat. Honour Daniel Thorburn. 29–35.
Email : kep[at]upnvj.ac.id
Whatsapp : 0821-1259-2258
Website : https://kep.upnvj.ac.id/